PINE LIBRARY
Atualizado ApproximateGaussianSmoothing
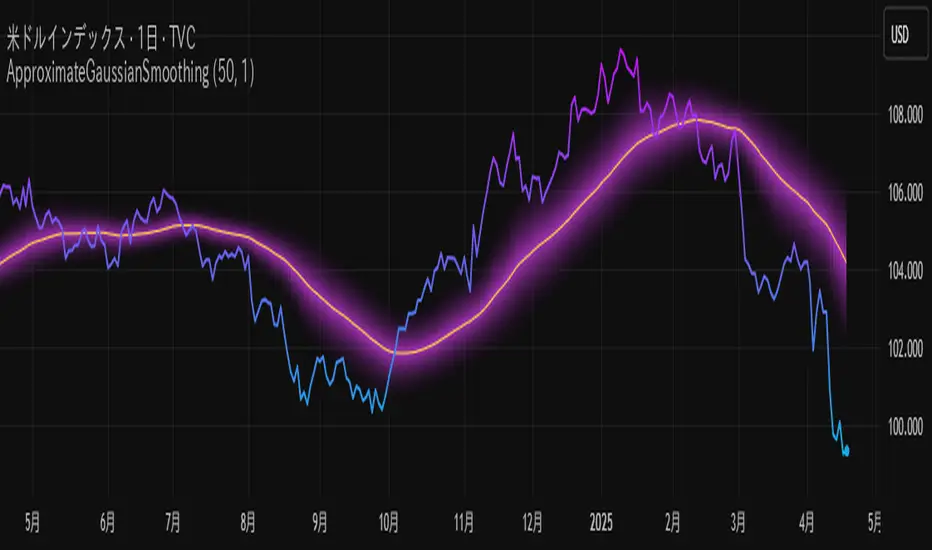
Library "ApproximateGaussianSmoothing"
This library provides a novel smoothing function for time-series data, serving as an alternative to SMA and EMA. Additionally, it provides some statistical processing, using moving averages as expected values in statistics.
'Approximate Gaussian Smoothing' (AGS) is designed to apply weights to time-series data that closely resemble Gaussian smoothing weights. it is easier to calculate than the similar ALMA.
In case AGS is used as a moving average, I named it 'Approximate Gaussian Weighted Moving Average' (AGWMA).
The formula is:
AGWMA = (EMA + EMA(EMA) + EMA(EMA(EMA)) + EMA(EMA(EMA(EMA)))) / 4
The EMA parameter alpha is 5 / (N + 4), using time period N (or length).
ma(src, length)
Calculate moving average using AGS (AGWMA).
Parameters:
src (float): Series of values to process.
length (simple int): Number of bars (length).
Returns: Moving average.
analyse(src, length)
Calculate mean and variance using AGS.
Parameters:
src (float): Series of values to process.
length (simple int): Number of bars (length).
Returns: Mean and variance.
analyse(dimensions, sources, length)
Calculate mean and variance covariance matrix using AGS.
Parameters:
dimensions (simple int): Dimensions of sources to process.
sources (array<float>): Series of values to process.
length (simple int): Number of bars (length).
Returns: Mean and variance covariance matrix.
trend(src, length)
Calculate intercept (LSMA) and slope using AGS.
Parameters:
src (float): Series of values to process.
length (simple int): Number of bars (length).
Returns: Intercept and slope.
This library provides a novel smoothing function for time-series data, serving as an alternative to SMA and EMA. Additionally, it provides some statistical processing, using moving averages as expected values in statistics.
'Approximate Gaussian Smoothing' (AGS) is designed to apply weights to time-series data that closely resemble Gaussian smoothing weights. it is easier to calculate than the similar ALMA.
In case AGS is used as a moving average, I named it 'Approximate Gaussian Weighted Moving Average' (AGWMA).
The formula is:
AGWMA = (EMA + EMA(EMA) + EMA(EMA(EMA)) + EMA(EMA(EMA(EMA)))) / 4
The EMA parameter alpha is 5 / (N + 4), using time period N (or length).
ma(src, length)
Calculate moving average using AGS (AGWMA).
Parameters:
src (float): Series of values to process.
length (simple int): Number of bars (length).
Returns: Moving average.
analyse(src, length)
Calculate mean and variance using AGS.
Parameters:
src (float): Series of values to process.
length (simple int): Number of bars (length).
Returns: Mean and variance.
analyse(dimensions, sources, length)
Calculate mean and variance covariance matrix using AGS.
Parameters:
dimensions (simple int): Dimensions of sources to process.
sources (array<float>): Series of values to process.
length (simple int): Number of bars (length).
Returns: Mean and variance covariance matrix.
trend(src, length)
Calculate intercept (LSMA) and slope using AGS.
Parameters:
src (float): Series of values to process.
length (simple int): Number of bars (length).
Returns: Intercept and slope.
Notas de Lançamento
v2更新:
trend(src, length)
Calculate trend statistics using AGS.
Parameters:
src (float): Series of values to process.
length (simple int): Number of bars (length).
Returns: Slope, intercept, correlation and RSS.
Notas de Lançamento
v3Notas de Lançamento
v4Add:
linreg(src1, src2, length)
Calculate linear regression using AGS.
Parameters:
src1 (float): Series of values to process.
src2 (float): Series of values to process.
length (simple int): Number of bars (length).
Returns: Slope, intercept and MSE.
correlation(src1, src2, length)
Calculate correlation using AGS.
Parameters:
src1 (float): Series of values to process.
src2 (float): Series of values to process.
length (simple int): Number of bars (length).
Returns: Correlation coefficient.
Delete:
trend(src, length)
Calculate trend statistics using AGS.
To get trend statistics, use the linreg method with bar_index as the first argument.
Biblioteca do Pine
No verdadeiro espirito do TradingView, o autor desse código Pine o publicou como uma biblioteca de código aberto, para que outros programadores Pine da nossa comunidade possam reusa-los. Parabéns ao autor! Você pode usar essa biblioteca privadamente ou em outras publicações de código aberto, mas a reutilização desse código em publicações é regida pelas Regras da Casa.
Aviso legal
As informações e publicações não devem ser e não constituem conselhos ou recomendações financeiras, de investimento, de negociação ou de qualquer outro tipo, fornecidas ou endossadas pela TradingView. Leia mais em Termos de uso.
Biblioteca do Pine
No verdadeiro espirito do TradingView, o autor desse código Pine o publicou como uma biblioteca de código aberto, para que outros programadores Pine da nossa comunidade possam reusa-los. Parabéns ao autor! Você pode usar essa biblioteca privadamente ou em outras publicações de código aberto, mas a reutilização desse código em publicações é regida pelas Regras da Casa.
Aviso legal
As informações e publicações não devem ser e não constituem conselhos ou recomendações financeiras, de investimento, de negociação ou de qualquer outro tipo, fornecidas ou endossadas pela TradingView. Leia mais em Termos de uso.